Hitting the sweet spot of complexity: Reasons why the development of new custom-tailored models is still warranted and should be encouraged in aquatic sciences
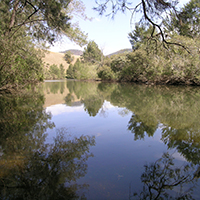
All claims expressed in this article are solely those of the authors and do not necessarily represent those of their affiliated organizations, or those of the publisher, the editors and the reviewers. Any product that may be evaluated in this article or claim that may be made by its manufacturer is not guaranteed or endorsed by the publisher.
Authors
Process-based aquatic ecosystem models are increasingly being developed and used in freshwater ecology and other aquatic sciences, as they are powerful tools to gain a mechanistic understanding of ecological processes and inform policy and decision making in environmental management. Over the last decades, not only have these models increased considerably in number, but also in their degree of complexity, which can improve predictive capacity. Nevertheless, it is also because of the higher degree of complexity of many models of current widespread use, that not all the hypotheses and assumptions upon which they have been built are always met by the relatively simple experiments that characterise fundamental ecological research. This is true for both laboratory experiments and those carried out outdoors, under semi-controlled conditions. Examples of the latter are the mesocosms experiments through which several novel questions are nowadays being addressed. In this article, we present our views on why the development of new custom-tailored aquatic ecosystem models of varying degrees of complexity is still very much warranted and should, therefore, be encouraged despite arguments in favour of always increasing complexity and against the creation of new models that are largely based on previously published ones (‘reinventing the wheel’). Deciding on the right complexity level should be linked to the biological organisation levels that are relevant to the specific research questions, and to how much knowledge on the subject is already available. Spatial and temporal scales are additional factors that a modeller should weigh in when deciding on the complexity of a model. To address these needs in the long term, the modelling community needs to grow. Training a new generation of model developers will not only benefit other scientists to better design future experiments but will also facilitate interdisciplinary research and teamwork, approaches such as ensemble modelling, as well as the communication of science to managers and many other stakeholders.
Supporting Agencies
Education, Audiovisual and Culture Executive Agency (EACEA) of the European CommissionHow to Cite

This work is licensed under a Creative Commons Attribution-NonCommercial 4.0 International License.